The rising threat of antibiotic resistance presents a critical challenge to global healthcare. Conventional antibiotics are becoming less effective, with multidrug-resistant bacteria and fungi endangering millions of lives annually. To address this crisis, researchers of some Chinese universities have turned to artificial intelligence (AI) as a tool for the de novo design of antimicrobial peptides (AMPs), an alternative class of antimicrobial agents that disrupt cell membranes and exhibit broad-spectrum activity. While existing AI models for AMP generation have shown promise, they often suffer from limited novelty and diversity. However, a groundbreaking study has introduced a novel AI-driven pipeline that leverages a latent diffusion model to generate and filter potent AMPs, pushing the boundaries of drug discovery..
The Urgent Need for Novel Antimicrobial Agents
Since their discovery, antibiotics have been a cornerstone of modern medicine. However, their widespread misuse has accelerated the emergence of drug-resistant pathogens. Experts predict that by 2050, antimicrobial resistance (AMR) could surpass cancer as the leading cause of death globally. AMPs offer a viable alternative due to their unique mode of action, which makes it more difficult for microbes to develop resistance. Despite their potential, AMP development has been hindered by the vast space in chemistry that still remains unexplored and by the traditional discovery methods that still remain expensive and time-consuming.
AI-Powered AMP Design: A Breakthrough Approach
The research team developed a novel AI pipeline that integrates latent diffusion models with molecular dynamics to generate diverse AMPs. Unlike previous generative models, this approach achieves a superior balance between diversity and accuracy. The pipeline consists of two key stages: generation and filtration. In the generative phase, a variational autoencoder (VAE) maps peptide sequences to latent variables, which are then used by the diffusion model to generate new sequences. The decoder reconstructs these sequences into potential AMPs. To ensure only the most promising AMPs are selected, a rigorous three-step filtering strategy is applied, incorporating classifier predictions, sequence clustering, and molecular dynamics simulations. This process refines the output by eliminating redundant or biologically nonviable sequences.
Experimental Validation: A Major Leap Forward
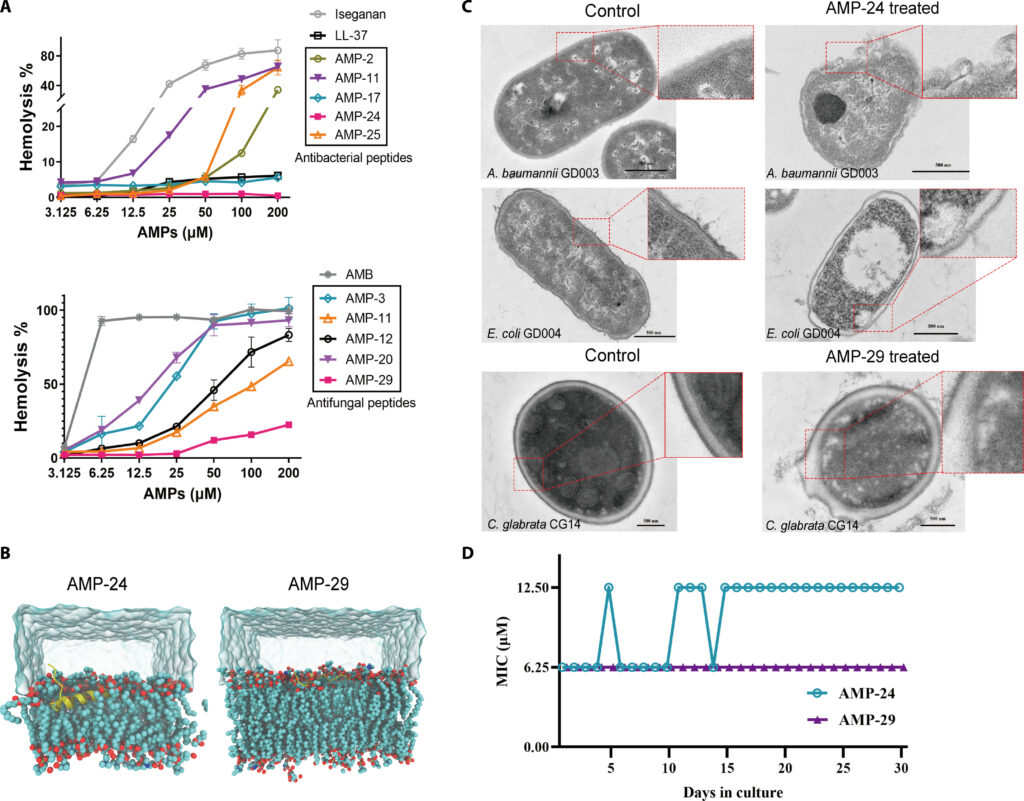
To validate the effectiveness of this AI-driven pipeline, researchers synthesized forty candidate peptides. Remarkably, twenty-five of these exhibited antibacterial or antifungal activity, demonstrating the model’s ability to generate highly effective AMPs. Among the most promising candidates were AMP-24 and AMP-29. AMP-24 exhibited potent antibacterial activity against Gram-negative pathogens, including Acinetobacter baumannii, a notorious multidrug-resistant bacterium. AMP-24 also demonstrated in vivo efficacy in both skin and lung infection models. AMP-29 showed selective antifungal activity against Candida glabrata, outperforming existing antifungal peptides in a murine skin infection model.
Understanding the Mechanism of Action
Further analysis revealed that these AMPs act by disrupting microbial membranes. Molecular dynamics simulations confirmed that AMP-24 binds to bacterial lipid membranes in a manner consistent with the toroidal pore model, leading to membrane destabilization and cell death. Similarly, AMP-29 effectively compromised fungal cell membranes, causing structural damage observable through transmission electron microscopy.
Addressing Safety and Resistance Concerns
While AMPs hold promise, their potential toxicity to human cells and the risk of resistance development are still critical concerns. The study conducted extensive hemolysis and cytotoxicity assays, demonstrating that AMP-24 and AMP-29 exhibit low toxicity levels, making them suitable candidates for further drug development. Additionally, prolonged exposure experiments revealed that these peptides had minimal potential to induce microbial resistance, a major advantage over conventional antibiotics.
Implications and Future Directions
The success of this AI-powered pipeline represents a significant milestone in antimicrobial drug discovery. Thanks to the continuing research of new AMPs and the continuing improvement of their selections, this approach has the potential to revolutionize the development of new antimicrobial agents. Moreover, the methodology can be adapted to design peptides targeting other diseases, including cancer and diabetes.
Despite these advances, challenges remain. Future iterations of the model could integrate additional parameters, such as secondary structures and physicochemical properties, to refine AMP generation further. Continued research and collaboration between AI specialists and microbiologists will be essential in translating these findings into clinically viable treatments.
Leave a Reply